Artificial Intelligence / Machine Learning
Curiosity May Be Vital for Truly Smart AI
Making machines inquisitive could improve their ability to perform important complex tasks.
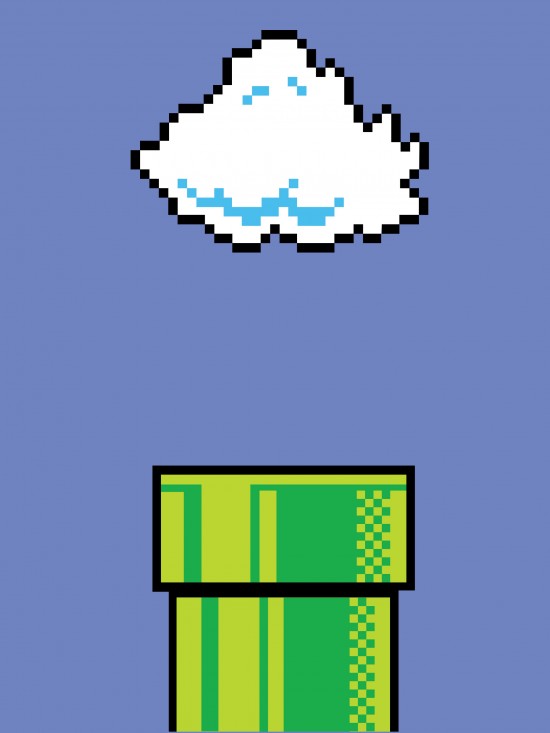
A computer algorithm equipped with a form of artificial curiosity can learn to solve tricky problems even when it isn’t immediately clear what actions might help it reach this goal.
Researchers at the University of California, Berkeley, developed an “intrinsic curiosity model” to make their learning algorithm work even when there isn’t a strong feedback signal. The curiosity model developed by this team sees the AI software controlling a virtual agent in a video game seek to maximize its understanding of its environment and especially aspects of that environment that affect it. There have been previous efforts to give AI agents curiosity, but these have tended to work in a more simplistic way.
The trick may help address a shortcoming of today’s most powerful machine-learning techniques, and it could point to ways of making machines better at solving real-world problems.
“Rewards in the real world are very sparse,” says Pulkit Agrawal, a PhD student at UC Berkeley who carried out the research with colleagues. “Babies do all these random experiments, and you can think of that as a kind of curiosity. They are learning some sort of skills.”
Several powerful machine-learning techniques have made machines smarter in recent years. Among these, a method known as reinforcement learning has made it possible for machines to accomplish things that would be difficult to define in code. Reinforcement learning involves using positive rewards to guide an algorithm’s behavior toward a particular goal (see “10 Breakthrough Technologies 2017: Reinforcement Learning”).
Reinforcement learning was a fundamental part of AlphaGo, a program developed by DeepMind, to play the abstract and complex board game Go with incredible skill. The technique is now being explored as a way to imbue machines with other skills that may be impossible to code manually. For instance, it can provide a way for a robot arm to work out for itself how to perform a desired chore.
Reinforcement learning has its limitations, though. Agrawal notes that it often takes a huge amount of training to learn a task, and the process can be difficult if the feedback required isn’t immediately available. For instance, the method doesn’t work for computer games in which the benefits of certain behaviors isn’t immediately obvious. That’s where curiosity could help.
The researchers tried the approach, in combination with reinforcement learning, within two simple video games: Mario Bros., a classic platform game, and VizDoom, a basic 3-D shooter title.
In both games, the use of artificial curiosity made the learning process more efficient. In the 3-D game, for instance, instead of spending an excessive amount of time bumping into walls, the agent moved around its environment, learning to navigate more quickly. Even without any other reward, the agent was able to navigate both games surprisingly well. In Mario Bros. it learned to avoid getting killed because this lessened its ability to explore and learn about its environment.
A paper describing the research will be published at a major AI conference later this year.
Artificial curiosity has been an active area of research for some time. Pierre-Yves Oudeyer, a research director at the French Institute for Research in Computer Science and Automation, has pioneered, over the past several years, the development of computer programs and robots that exhibit simple forms of inquisitiveness.
“What is very exciting right now is that these ideas, which were very much viewed as ‘exotic’ by both mainstream AI and neuroscience researchers, are now becoming a major topic in both AI and neuroscience,” Oudeyer says.
The work could have real practical benefits. The UC Berkeley team is keen to test it on robots that use reinforcement learning to work out how to do things like grasps awkward objects. Agrawal says robots can waste a huge amount of time performing random gestures. When equipped with innate curiosity, such a robot should more quickly explore its surroundings and experiment with nearby objects, he says.
Brenden Lake, a research scientist at New York University who builds computational models of human cognitive capabilities, says the work seems promising. “Developing machines with similar qualities is an important step toward building machines that learn and think like people,” he said in an e-mail. “It’s very impressive that by using only curiosity-driven learning, the agent can learn to navigate a level in Mario. The agent doesn’t even look at the game score.”
At the same time, says Lake, the inquisitiveness demonstrated by the new program is actually pretty different from, say, that of a child. Humans tend to display a much deeper interest in their world, he says.
“It’s a very egocentric form of curiosity,” Lake says. “The agent is only curious about features of its environment that relate to its own actions. People are more broadly curious. People want to learn about the world in ways less directly tied to their own actions.”