Artificial Intelligence
“We’re in a diversity crisis”: cofounder of Black in AI on what’s poisoning algorithms in our lives
Timnit Gebru looks around the AI world and sees almost no one who looks like her. That’s a problem for all of us.
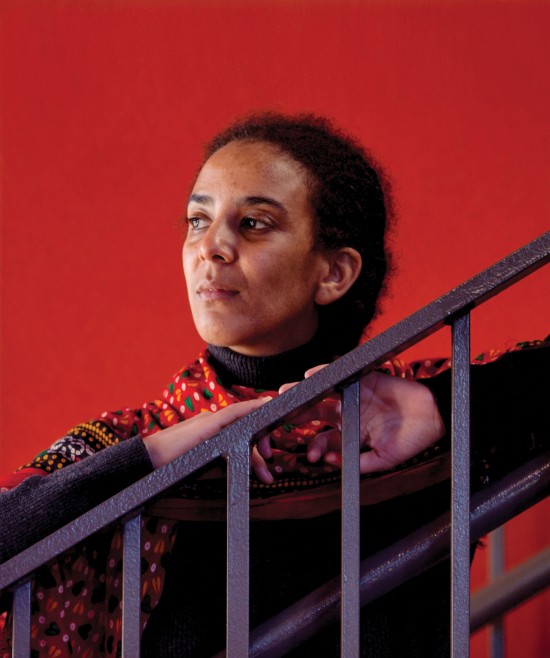
Artificial intelligence is an increasingly seamless part of our everyday lives, present in everything from web searches to social media to home assistants like Alexa. But what do we do if this massively important technology is unintentionally, but fundamentally, biased? And what do we do if this massively important field includes almost no black researchers? Timnit Gebru is tackling these questions as part of Microsoft’s Fairness, Accountability, Transparency, and Ethics in AI group, which she joined last summer. She also cofounded the Black in AI event at the Neural Information Processing Systems (NIPS) conference in 2017 and was on the steering committee for the first Fairness and Transparency conference in February. She spoke with MIT Technology Review about how bias gets into AI systems and how diversity can counteract it.
How does the lack of diversity distort artificial intelligence and specifically computer vision?
I can talk about this for a whole year. There is a bias to what kinds of problems we think are important, what kinds of research we think are important, and where we think AI should go. If we don’t have diversity in our set of researchers, we are not going to address problems that are faced by the majority of people in the world. When problems don’t affect us, we don’t think they’re that important, and we might not even know what these problems are, because we’re not interacting with the people who are experiencing them.
Are there ways to counteract bias in systems?
The reason diversity is really important in AI, not just in data sets but also in researchers, is that you need people who just have this social sense of how things are. We are in a diversity crisis for AI. In addition to having technical conversations, conversations about law, conversations about ethics, we need to have conversations about diversity in AI. We need all sorts of diversity in AI. And this needs to be treated as something that’s extremely urgent.
From a technical standpoint, there are many different kinds of approaches. One is to diversify your data set and to have many different annotations of your data set, like race and gender and age. Once you train a model, you can test it out and see how well it does by all these different subgroups. But even after you do this, you are bound to have some sort of bias in your data set. You cannot have a data set that perfectly samples the whole world.
Something I’m really passionate about and I’m working on right now is to figure out how to encourage companies to give more information to users or even researchers. They should have recommended usage, what the pitfalls are, how biased the data set is, etc. So that when I’m a startup and I’m just taking your off-the-shelf data set or off-the-shelf model and incorporating it into whatever I’m doing, at least I have some knowledge of what kinds of pitfalls there may be. Right now we’re in a place almost like the Wild West, where we don’t really have many standards [about] where we put out data sets.
And then there are just some things you probably shouldn’t be using machine learning for right now, and we don’t have a clear guideline for what those things are. We should say that if you’re going to use machine learning for this particular task, the accuracy of your model should be at least X, and it should be fair in this particular respect. We don’t have any sort of guidelines for that either. AI is just now starting to be baked into the mainstream, into a product everywhere, so we’re at a precipice where we really need some sort of conversation around standardization and usage.
What’s been the driving motivation behind your work with Google Street View and other demographic research?
At the time we started this project, there was very little work being done to try to analyze culture using images. But we know that online, most of our data is in the form of images. One of our motivations was to show that you could do social analyses using images.
This could be very useful in cases where getting survey-based data is really hard. There are places in the world where the infrastructure is not there and the resources are not there to send people door to door and gather [census] data, [but where] having an understanding of the different types of populations that live in your country would be very helpful.
But then again, this is exactly the thing that also made me want to study fairness. Because if I’m going to be continuing to do this line of work, I really need to have a better understanding of the potentially negative repercussions. What are the repercussions for surveillance? Also, what are the repercussions for a data-set bias? In any sort of data-mining project, you’re going to have a bias. So my line of work there was really what led me to want to spend some time in the fairness community to understand where the pitfalls could be.
What issues are you hoping to address with this first Fairness and Transparency conference?
This is really the first conference that is addressing the issues of fairness, accountability, ethics, and transparency in AI. There have been workshops at other conferences, and mostly there have been workshops at either natural-language-processing-based conferences or machine-learning-based conferences. It’s really important to have the stand-alone conference because it needs to be worked on by people from many disciplines who talk to each other.
Machine-learning people on their own cannot solve this problem. There are issues of transparency; there are issues of how the laws should be updated. If you’re going to talk about bias in health care, you want to talk to [health-care professionals] about where the potential biases could be, and then you can think about how to have a machine-learning-based solution.
What has been your experience working in AI?
It’s not easy. I love my job. I love the research that I work on. I love the field. I cannot imagine what else I would do in that respect. That being said, it’s very difficult to be a black woman in this field. When I started Black in AI, I started it with a couple of my friends. I had a tiny mailing list before that where I literally would add any black person I saw in this field into the mailing list and be like, “Hi, I’m Timnit. I’m black person number two. Hi, black person number one. Let’s be friends.”
What really just made it accelerate was [in 2016] when I went to NIPS and someone was saying there were an estimated 8,500 people. I counted six black people. I was literally panicking. That’s the only way I can describe how I felt. I saw that this field was growing exponentially, hitting the mainstream; it’s affecting every part of society. At the same time, I also saw a lot of rhetoric about diversity and how a lot of companies think it’s important.
And I saw a mismatch between the rhetoric and action. Because six black people out of 8,500—that’s a ridiculous number, right? That is almost zero percent. I was like, “We have to do something now.” I want to give a call to action to people who believe diversity is important. Because it is an emergency, and we have to do something about it now.