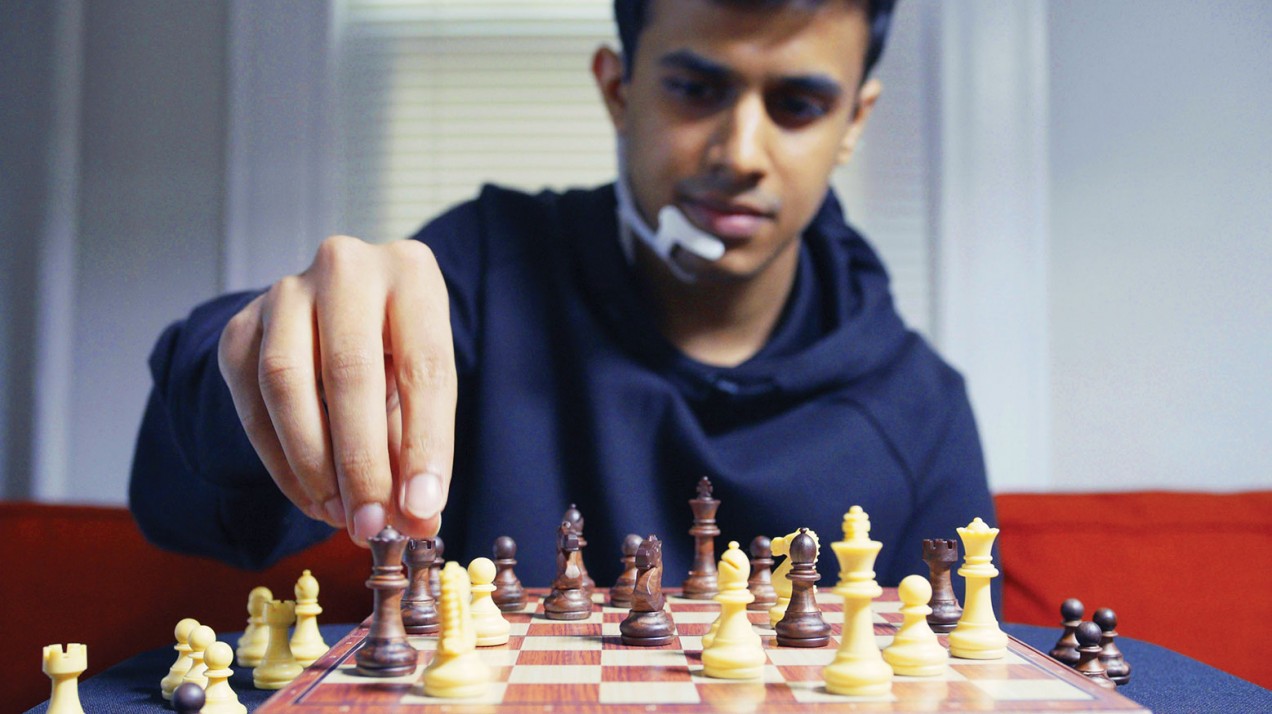
MIT researchers have developed a computer interface that can transcribe words the user verbalizes internally but does not actually speak aloud.
Electrodes in the wearable device pick up neuromuscular signals in the jaw and face that are triggered by saying words “in your head” but are undetectable to the human eye. The signals are fed to a machine-learning system that has been trained to correlate particular signals with particular words.
The device, called AlterEgo, also includes bone-conduction headphones, which transmit vibrations through facial bones to the inner ear. Because the headphones don’t obstruct the ear canal, the system can convey information without interrupting conversation or interfering with the auditory experience.
AlterEgo provides a private and discreet channel for transmitting and receiving information, letting wearers do such things as undetectably pose and receive answers to difficult computational problems or silently report opponents’ moves in a chess game and just as silently receive computer--recommended responses.
“We basically can’t live without our cell phones,” says Pattie Maes, a professor of media arts and sciences and thesis advisor for Arnav Kapur, the Media Lab graduate student who led the system’s development. “But at the moment, the use of those devices is very disruptive. If I want to look something up that’s relevant to a conversation I’m having, I have to find my phone and type in the passcode and open an app and type in some search keyword.” The goal with AlterEgo was to build a noninvasive intelligence augmentation system that would be completely controlled by the user.
The idea that internal verbalizations have physical correlates has been around since the 19th century, and it was seriously investigated in the 1950s. One aim of the speed-reading movement of the 1960s was to eliminate this “subvocalization,” as it’s known.
But subvocalization as a computer interface is largely unexplored. To determine which facial locations provide the most reliable neuromuscular signals, the researchers attached 16 electrodes to the research subjects’ faces and had them subvocalize the same series of words four times.
The researchers wrote code to analyze the resulting data and found that signals from seven electrode locations were consistently able to distinguish subvocalized words. In a paper they presented at the Association for Computing Machinery’s ACM Intelligent User Interface conference, they described a prototype of a wearable silent-speech interface, which wraps around the back of the neck like a telephone headset and has tentacle-like curved appendages that touch the face at seven locations on either side of the mouth and along the jaws.
But in subsequent experiments, the researchers achieved comparable results using only four electrodes along one jaw, which could make for a less obtrusive device.
Having selected the electrode locations, the researchers collected data on a few computational tasks with vocabularies of about 20 words each. One was arithmetic, in which the user subvocalized large addition or multiplication problems; another was the chess application, in which the user reported moves using the standard chess numbering system.
Then, for each application, they used a neural network to find correlations between particular neuromuscular signals and particular words.
Using the prototype interface, the researchers conducted a usability study in which 10 subjects spent about 15 minutes customizing the arithmetic application to their own neurophysiology and another 90 minutes using it to execute computations. In that study, transcription accuracy averaged about 92 percent. But, Kapur says, performance should improve with more training data, which could be collected during ordinary use.
In ongoing work, the researchers are collecting data on more elaborate conversations, in the hope of building applications with much more expansive vocabularies. Says Kapur, “I think we’ll achieve full conversation someday.”